Machine Learning Odyssey: Charting Unexplored Terrains in Bug Bounty Systems

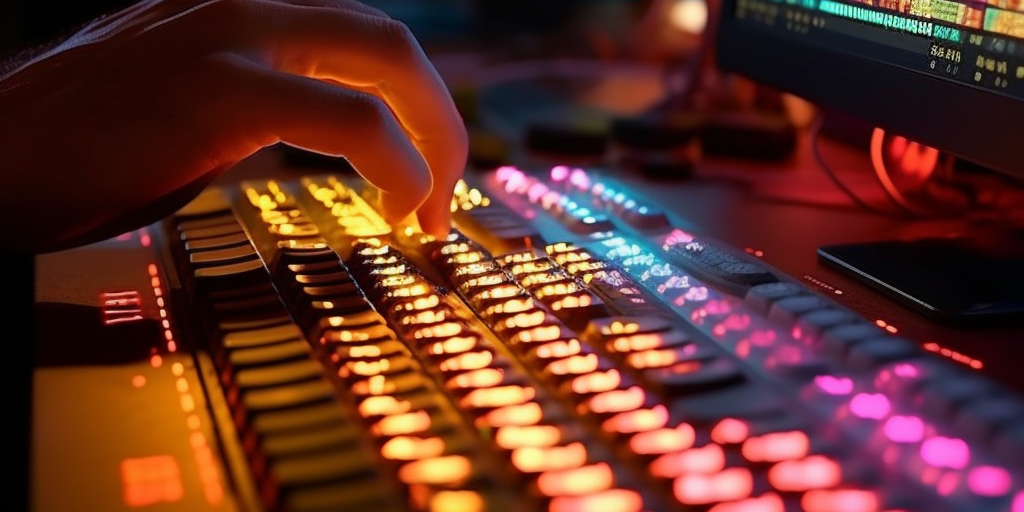

Unable to resist the cloud: Reflecting on my “Bug Bounty Backend” infrastructure
As I sit down today, working on my project – a bug bounty system – my mind is brimming with ideas, strategies, and potential paths to follow. My journey began with an insightful article by Ryan Elkins on his website, Brevity in Motion. Elkins' work, particularly his Project Straylight series, has been an eye-opening exploration into the vast landscape of external IP domain reconnaissance and attack surface visualization. His methods of retrieving extensive raw DNS data and converting it into comprehensible visual information have provided an intriguing starting point for my project.
After hours of meticulous exploration and learning, I found myself facing the daunting task of developing my application. The first question that popped into my mind was, "What's the best approach to take?" Should I hand-write schemas and transform them into serverless API functions? Or is there a more efficient, less time-consuming method?
AWS Sagemaker
The answer came to me in the form of AWS SageMaker, a machine learning platform that I came across while poring over images in Elkins' article. The more I delved into AWS SageMaker, the more intrigued I became. Its features – notebooks, repositories, buckets, EC2s, and Lambda functions – seemed to check all the boxes for my project's needs. Its other features like SageMaker Autopilot, Batch Transform, and SageMaker Clarify further enhance its capabilities, offering users with minimal machine learning knowledge the ability to build sophisticated models quickly and easily.
A specific tutorial on SageMaker Studio struck me profoundly (though I'm unable to provide specific details about this tutorial due to technical constraints). After watching it, I felt a rush of inspiration, a sense of clarity. It suddenly appeared to me that this could be the perfect platform to build my bug bounty system and Langchain integration.
Reflecting on the past year, I can't help but feel that every step I've taken, every challenge I've overcome, and every lesson I've learned, was all leading up to this moment. It's as if my journey was designed to equip me with the knowledge and skills I needed to embark on this exciting project.
I am thrilled about the potential of integrating machine learning into my project through AWS SageMaker. Yet, I am also humbled by the vastness of the uncharted territory that lies before me. Where will this path lead me? I can't say for sure. But one thing I do know is that the process of building my knowledge while simultaneously constructing my infrastructure has been an enriching and rewarding experience.
As I continue on this journey, I look forward to the challenges, discoveries, and innovations that lie ahead. And I can't wait to share them with you. Stay tuned!